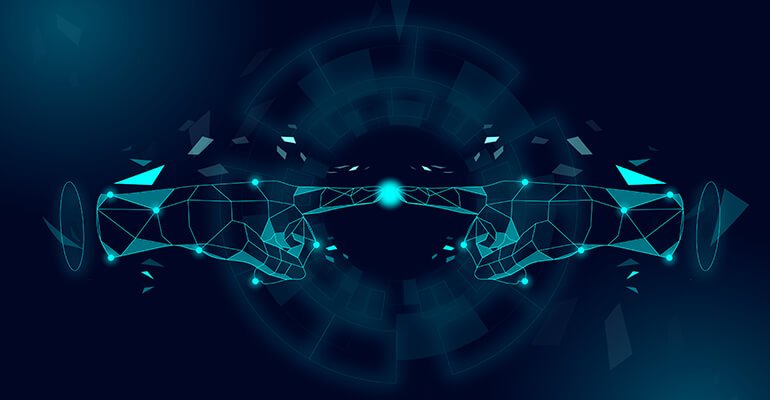
What are actually AI and ML?
Throughout recent years, the topic of AI and ML is getting hotter and hotter. Although we’re focused on publications related to innovation in Market Research in our blog, we want to step back and take a look at the bigger picture.
The year 2020 is exceptional, not only because of the tremendous shift in the human mind and behavior due to COVID crisis development. This year also reshaped many industries and jobs, demanding automation and optimization in many of them.
At the same time, AI and ML technologies are progressing fast. One particular event of interest is the June 2020 release of the GPT-3 Autoregressive Transformer language model (by OpenAI), capable of producing human-like text. And while the code of the model is not yet available publicly, the possibilities that GPT-3 opens in the form of humanizing the AI technology is astonishing.
But, what are AI and ML? Many people are using these two terms altogether or as synonyms; however, they mean different things.
AI. Artificial intelligence is a technology that enables the creation of systems that can simulate human intelligence. As the abbreviation states, this is human-made thinking power. The AI does not require pre-programming and uses machine learning algorithms and deep learning neural networks to reinforce and fine-tune the learned. Such algorithms allow the AI to work with its own intelligence. Thus, AI has a vast range of scope, and its fundamental goal is to maximize the chances of success. AI is used in human-like activities like assistance (Siri, Alexa), chatbots, online gaming, and the creation of humanoid robots. AI can work with unstructured data as well. Back to GPT-3 – it can write essays and tell jokes.
ML. Machine learning is a part sub-field of artificial intelligence, enabling machines to learn from past data or experiences without being explicitly programmed. It supports AI with its models. It allows a computer system to make predictions or make decisions that result from processing huge amounts of structured or semi-structured data. ML is all about accuracy and finding patterns. When new data is introduced in an ML model, the model changes, but it lacks reasoning, which is typical for AI. From this perspective, the ML has a very limited domain, and its purpose is to create tools focused on performing well tasks they are trained for. So, if, an example, you train an ML model to detect English gibberish text, it will do precisely this and will be limited to the scope of the particular language. ML is a good fit for search algorithms, market predictions, email spam detection, and other text, audio, and visual processing.
But at the end of the day, whatever the technology, the most important is not the technology itself. An example, even the most precise machine learning models don’t create value on their own. The value hides in the context of the products that they power. Thus the most challenging task – to figure out how to use ML in a product in the most efficient way and how to improve it daily. We know this firsthand, as we are using both AI with deep learning neural networks and ML for training and reinforcing models in some of our tools.